DECISION MAKING UNDER UNCERTAINTY
SHOULD YOU TAKE AN UMBRELLA?
Until now, we’ve been a little imprecise about what judgment actually is.
To explain it, we introduce a decision-making tool: the decision tree (2). It is especially useful for decisions under uncertainty, when you are not sure what will happen if you make a particular choice.
Let’s consider a familiar choice you might face. Should you carry an umbrella on a walk? You might think that an umbrella is a thing you hold over your head to stay dry, and you’d be right. But an umbrella is also a kind of insurance, in this case, against the possibility of rain. So, the following framework applies to any insurance-like decision to reduce risk.
Clearly, if you knew it was not going to rain, you would leave the umbrella at home. On the other hand, if you knew it would rain, then you would certainly take it with you. In figure 7-2, we represent this using a tree-like diagram. At the root of the tree are two branches representing the choices you could make: “leave umbrella” or “take umbrella.” Extending from these are two branches that represent what you are uncertain about: “rain” versus “shine.” Absent a good weather forecast, you do not know. You might know that, at this time of the year, sun is three times more likely than rain. This would give you a three-quarters chance of sun and a one-quarter chance of rain. This is your prediction. Finally, at the tips of the branches are the consequences. If you don’t take an umbrella and it rains, you get wet, and so on.

What decision should you make? This is where judgment comes in. Judgment is the process of determining the reward to a particular action in a particular environment. It is about working out the objective you’re actually pursuing. Judgment involves determining what we call the “reward function”, the relative rewards and penalties associated with taking particular actions that produce particular outcomes. Wet or dry? Burdened by carrying an umbrella or unburdened?
Let’s assume that you prefer being dry without an umbrella (you rate it a 10 out of 10) more than being dry, but carrying an umbrella (8 out of 10) more than being wet (a big, fat 0). (See figure 7-3.) This gives you enough to act. With the prediction of rain one-quarter of the time and the judgment of the payoffs to being wet or carrying an umbrella, you can work out your average payoff from taking versus leaving the umbrella. Based on this, you are better off taking the umbrella (an average payoff of 8) than leaving it (an average payoff of 7.5) (3).

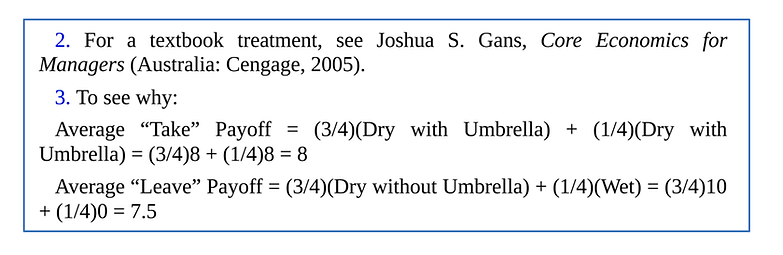
If you really hate toting an umbrella (a 6 out of 10), your judgment about preferences can also be accommodated. In this case, the average payoff from leaving an umbrella at home is unchanged (at 7.5), while the payoff from taking one is now 6. So, such umbrella haters will leave the umbrella at home.
This example is trivial: of course, people who hate umbrellas more than getting wet will leave them home. But the decision tree is a useful tool for figuring out payoffs for nontrivial decisions, too, and that is at the heart of judgment. Here, the action is taking the umbrella, the prediction is rain or shine, the outcome is whether you get wet, and judgment is anticipating happiness you will feel (“payoff”) from being wet or dry, with or without an umbrella. As prediction becomes better, faster, and cheaper, we’ll use more of it to make more decisions, so we’ll also need more human judgment and thus the value of human judgment will go up.
Em relação ao problema do guarda-chuva acima, podemos incrementá-lo ainda mais pensando no seguinte:
a) Se a quantidade de chuva for pequena e houver uma possibilidade de pegarmos um guarda-chuva, caso necessário, com um amigo que mora no meio do caminho entre a nossa casa e o trabalho.
b) outra opção que podemos pensar é: caso chova podemos esperar numa cafeteria perto do trabalho a chuva passar, dado que não temos nenhuma reunião agendada para essa manhã.
Em resumo: existem opções de planos B, C e D???
Seguem abaixo algumas explicações básicas do GPT-4 sobre Análise de Opções Reais.
**********************************************************
Real options analysis is a financial modeling technique used to assess and manage the potential value and risk associated with investment opportunities. It's particularly useful in situations where the future is uncertain and the decision to invest can be delayed or modified in response to new information. The term "real options" is derived from financial options in securities markets, but it applies these concepts to real-world assets and investment opportunities.
Here are the key aspects of real options analysis:
1. Flexibility and Decision Making: Real options analysis provides a framework to evaluate the flexibility an investor has to adapt their strategies based on how future scenarios unfold. This could involve delaying, expanding, contracting, or abandoning a project.
2. Types of Real Options: Common types include the option to defer (waiting for more information before investing), the option to expand (investing additional resources if the project goes well), the option to contract or abandon (reducing scale or exiting a project if it's performing poorly), and the option to switch (changing the project's strategy or output in response to market conditions).
3. Valuation Techniques: Real options analysis uses various valuation methods, often derived from financial options theory, such as the Black-Scholes model and binomial options pricing models. These methods help quantify the value of flexibility and the ability to make future investment decisions.
4. Applications: It's widely used in capital-intensive industries, such as oil and gas, mining, pharmaceuticals, and real estate development, where future market conditions and project outcomes are highly uncertain, and the decisions involve significant expenditures that are at least partially reversible or adjustable.
5. Advantages over Traditional NPV: While the traditional net present value (NPV) approach to investment appraisal considers a project's expected cash flows and the time value of money, it may not adequately account for the value of management flexibility in the face of uncertainty. Real options analysis attempts to address this limitation by valuing options that the management has to alter the course of the project in response to new information.
6. Challenges: Implementing real options analysis can be complex and requires detailed modeling of potential future scenarios. It also depends on accurate estimation of volatility and other parameters, which can be difficult in practice.
Overall, real options analysis is a powerful tool that adds a layer of strategic thinking to the financial evaluation of investments, capturing the value of flexibility and the ability to respond to new information in uncertain environments.
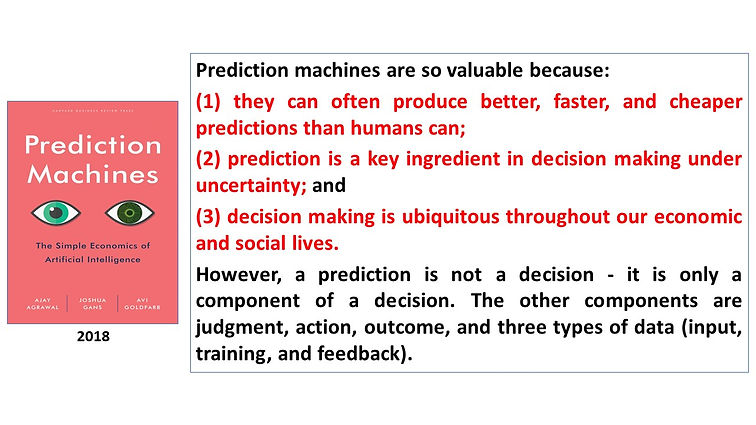
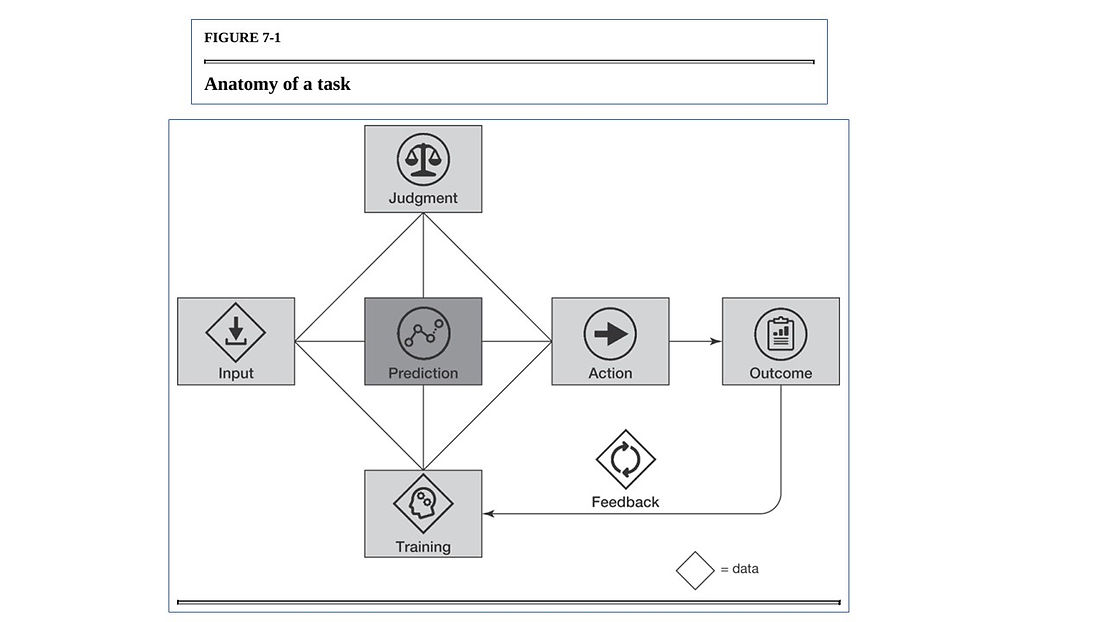


Setting the Stage (Chapter 2 - AI’s System Future)
In the next chapter, we revisit a theme from Prediction Machines—that advances in modern AI are, at their essence, an improvement in prediction technology. Moreover, predictions have value only as inputs into decision-making. Thus, we modify the previous definitions for the purposes of this book:
AI POINT SOLUTION: A prediction is valuable as a point solution if it improves an existing decision and that decision can be made independently.
AI APPLICATION SOLUTION: A prediction is valuable as an application solution if it enables a new decision or changes how a decision is made and that decision can be made independently.
AI SYSTEM SOLUTION: A prediction is valuable as a system solution if it improves existing decisions or enables new decisions, but only if changes to how other decisions are made are implemented.
For other technologies, while we have the benefit of hindsight to tell us exactly what was independent and what was dependent, with AI we must still figure out those aspects of the system. This book is about how to find them.
System Change Is Disruptive (Chapter 2 - AI’s System Future)
Prediction was a large driver of farmers’ key decisions: fertilization, seeding, and harvesting. The goal of these decisions was nearly universal - to maximize yield: “Farming had always involved judgment calls that turned on the instincts of the farmer. The Climate Corporation (https://climate.com/) had turned farming into decision science, and a matter of probabilities. The farmer was no longer playing roulette but blackjack. And David Friedberg was helping him to count the cards.”
Farmers were used to seeing technological change in the form of new tools they could use, but this knowledge was replacing how they made decisions. Indeed, the decisions themselves had not only changed but physically moved. Where? To San Francisco, far from rural America. This urban West Coast corporation was now telling farmers in Kansas that they should no longer be growing corn.
The Climate Corporation does not currently deal with all farming decisions. The farmer still makes some critical decisions. However, as Friedberg notes, “over time that’ll go to zero. Everything will be observed. Everything will be predicted”. Farmers are embracing this bit by bit. Author Michael Lewis recounts, “[N]o one ever asked Friedberg the question: If my knowledge is no longer useful, who needs me?” In other words, the portends are toward disruption and centralizing farm management. We don’t know how long it will take and whether some decisions cannot be automated. We do know that the industry sees high potential in these tools. Monsanto acquired the Climate Corporation in 2013 for $1.1 billion.
Step by step, as prediction machines improve, farmers are not simply taking those predictions and making decisions but ceding those decisions to others. This likely makes farm management better, as people with the right information, skills, incentives, and ability to coordinate increasingly make the decisions. But at the same time, what will the farmer’s role be? They are the landowners, but how long before that too changes?
The Plan for the Book (Chapter 2 - AI’s System Future)
In part 5 (book referenced below), we dig into the mechanism by which prediction can change who holds power, that is, how AI disrupts. We explain how AI adoption involves the decoupling of prediction and judgment that were previously bundled together in how decision-makers without a prediction machine at their disposal made decisions. This raises the question whether the current decision-maker is actually best positioned to supply that judgment. We then turn to who those judges might be, following decoupling. In particular, we explore how judgment can move from being decentralized to being at scale with a consequent concentration of power. Similarly, when prediction involves a change from a rule to a decision and then to a new system, new people have a role in decision-making and therefore become the new locus of power.
Complementing Prediction (Chapter 3 - AI Is Prediction Technology)
Predictions are not the only input into decision-making. o understand how prediction matters, it is necessary to understand two other key inputs into decisions: judgment and data. Judgment is best explained with an example. In the movie I, Robot, homicide detective Del Spooner lives in a future where robots serve humans. The detective hates robots, and that hatred drives much of the plot. The movie provides the backstory behind Spooner’s animosity toward robots.
Spooner’s car is in an accident with another car carrying a twelve-year-old girl; the cars veer off a bridge, and both the detective and the girl are clearly about to drown. A robot saves the detective, not the girl. He thinks the robot should have saved the girl, so he carries a grudge against robots.
Because it was a robot, Spooner could audit its decision. He learns that the robot predicted that he had a 45 percent chance of survival and that the girl only had an 11 percent chance. Therefore, given the robot had time to save only one person, the robot saved him. Spooner thinks that 11 percent was more than enough chance to try to save the girl instead, and a human being would have known that.
Maybe. That is a statement about judgment—the process of determining the reward to a particular action in a particular environment. If saving the girl is the right decision, then we can infer he believes the girl’s life is worth more than four times his. If she had an 11 percent chance of survival and he had a 45 percent chance, a human with that information who was forced to make the choice would have to specify the relative value of their lives. The robot was apparently programmed to judge all human lives to be of equal value. When using a prediction machine, we need to be explicit about judgment.
KEY POINTS (Chapter 3 - AI Is Prediction Technology)
1 - Recent advances in AI have caused a drop in the cost of prediction. We use prediction to take information we have (e.g., data on whether past financial transactions were fraudulent) and generate data we need but don’t have (e.g., whether a current financial transaction is fraudulent). Prediction is an input to decision-making. When the cost of an input falls, we use more of it. So, as prediction becomes cheaper, we will use more AI. As the cost of prediction falls, the value of substitutes for machine prediction (e.g., human prediction) will fall. At the same time, the value of complements to machine prediction will rise. Two of the main complements to machine prediction are data and judgment. We use data to train AI models. We use judgment along with predictions to make decisions. While prediction is an expression of likelihood, judgment is an expression of desire—what we want. So, when we make a decision, we contemplate the likelihood of each possible outcome that could arise from that decision (prediction) and how much we value each outcome (judgment).
2 - Perhaps the greatest misuse of AI predictions is treating the correlations they identify as causal. Often, correlations are good enough for an application. However, if we need AI to inform a causal relationship, then we use randomized experiments to collect the relevant data. These experiments are the best tool for statisticians to discover what causes what.
The Judgment Opportunity (Chapter 13 - A Great Decoupling)
Decoupling prediction and judgment creates opportunity. It means that who makes the decision is driven not by who does prediction and judgment best as a bundle, but who is best to provide judgment utilizing AI prediction. Once the AI provides the prediction, then the people with the best judgment can shine.
As we’ve noted, conceptually and increasingly as a matter of practice, AI is able to conduct predictions with a greater degree of precision than many radiologists. While it depends on what precisely is being predicted, in effect, an AI can be trained not by observing the predictions of radiologists but by matching the images to observed, reliable outcomes —for example, did pathology find a malignant tumor? Thus, AI prediction has the potential to become superior to human prediction, so much so that Vinod Khosla, a technology pioneer and well-known investor in AI, suggests that in the future it may be malpractice for radiologists not to rely on AI prediction.
Herein lies the issue—what would AI prediction do to the value of a radiologist’s judgment? Given the way radiologists (at least in the United States) operate, they are largely divorced from other information about the patient. Thus, if an AI predicts that a particular patient has a malignant tumor with 30 percent probability, under what imaginable circumstances could a medical system accept the judgment of one radiologist that the patient should be diagnosed and treated for the tumor versus that of another that they should not? Indeed, it is hard to imagine. Instead, one suspects that some committee of medical professionals will deliberate and debate the rules for diagnosis in advance of any machine prediction, and then that committee’s judgment would be subsequently applied at scale. The radiologist’s decision becomes decoupled into a machine prediction and a committee’s judgment.
Thinking in Bets (Chapter 14 - Thinking Probabilistically)
Thinking in bets requires you to recognize that predictions are uncertain, and to understand that the outcomes you experience are partly determined by luck. And that isn’t easy.
For cars, before self-driving, prediction and judgment rested with the driver. If a human driver hit a pedestrian, we never knew whether they made a prediction error (they thought the likelihood of hitting the person was effectively zero, so they did not brake) or a judgment error (they were in a rush and put a higher weight on getting to their destination quickly than avoiding an accident). If they had an accident, we assume their judgment was fine, but they made a mechanical error in generating their prediction of a collision. At present, society seems OK with this.
When you are designing a self-driving car, you can measure prediction error. But then you have to quantify judgment, which involves doing unpleasant things like calculating the cost of life and comparing that with the experience of being a passenger in a car (stopping too frequently from an abundance of caution is unpleasant). People have to trade this off implicitly all the time but demur when asked to be explicit. It will be no less unpleasant for an engineering and, perhaps, ethics team determining what to do regarding a self-driving car.
IDEIAS de Kahneman & Tversky
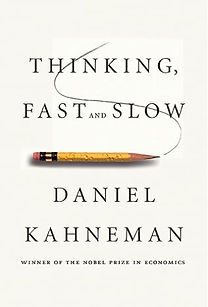
The book is divided into five parts.
Part 1 - Presents the basic elements of a two systems approach to judgment and choice. It elaborates the distinction between the automatic operations of System 1 and the controlled operations of System 2, and shows how associative memory, the core of System 1, continually constructs a coherent interpretation of what is going on in our world at any instant. I attempt to give a sense of the complexity and richness of the automatic and often unconscious processes that underlie intuitive thinking, and of how these automatic processes explain the heuristics of judgment.
Part 2 - Updates the study of judgment heuristics and explores a major puzzle: Why is it so difficult for us to think statistically? We easily think associatively, we think metaphorically, we think causally, but statistics requires thinking about many things at once, which is something that System 1 is not designed to do.
Part 3 - The difficulties of statistical thinking contribute to the main theme of Part 3, which describes a puzzling limitation of our mind: our excessive confidence in what we believe we know, and our apparent inability to acknowledge the full extent of our ignorance and the uncertainty of the world we live in. We are prone to overestimate how much we understand about the world and to underestimate the role of chance in events. Overconfidence is fed by the illusory certainty of hindsight. My views on this topic have been influenced by Nassim Taleb, the author of The Black Swan.
Part 4 - The focus is a conversation with the discipline of economics on the nature of decision making and on the assumption that economic agents are rational. This section of the book provides a current view, informed by the two-system model, of the key concepts of prospect theory, the model of choice that Amos and I published in 1979. Subsequent chapters address several ways human choices deviate from the rules of rationality.
Part 5 - Describes recent research that has introduced a distinction between two selves, the experiencing self and the remembering self, which do not have the same interests. How two selves within a single body can pursue happiness raises some difficult questions, both for individuals and for societies that view the well-being of the population as a policy objective.
Daniel Kahneman, “Thinking, Fast and Slow”, Farrar, Straus and Giroux, 1st edition (October 1, 2011).
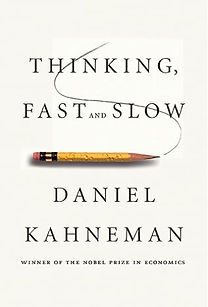
PART 4
Chapter 25 - Bernoulli’s Errors
Chapter 26 - Prospect Theory
Blind Spots of Prospect Theory
Chapter 29 - The Fourfold Pattern
Allais’s Paradox
The Fourfold Pattern
Chapter 31 - Risk Policies
Chapter 32 - Keeping Score
Chapter 25 - Bernoulli’s Errors
Bernoulli’s essay is a marvel of concise brilliance. He applied his new concept of expected utility (which he called “moral expectation”) to compute how much a merchant in St. Petersburg would be willing to pay to insure a shipment of spice from Amsterdam if “he is well aware of the fact that at this time of year of one hundred ships which sail from Amsterdam to Petersburg, five are usually lost.”
Bernoulli also offered a solution to the famous “St. Petersburg paradox,” in which people who are offered a gamble that has infinite expected value (in ducats) are willing to spend only a few ducats for it.
Chapter 26 - Prospect Theory
His theory (Bernoulli’s model) is too simple and lacks a moving part. The missing variable is the reference point, the earlier state relative to which gains and losses are evaluated. In Bernoulli’s theory you need to know only the state of wealth to determine its utility, but in prospect theory you also need to know the reference state
Although Amos and I were not working with the two-systems model of the mind, it’s clear now that there are three cognitive features at the heart of prospect theory: a) Evaluation is relative to a neutral reference point, which is sometimes referred to as an “adaptation level.”; b) A principle of diminishing sensitivity applies to both sensory dimensions and the evaluation of changes of wealth; and c) The third principle is loss aversion.
Blind Spots of Prospect Theory
Prospect theory and utility theory also fail to allow for regret.
Chapter 29 - The Fourfold Pattern
The improvement from 95% to 100% is another qualitative change that has a large impact, the certainty effect. Outcomes that are almost certain are given less weight than their probability justifies.
Because of the possibility effect, we tend to overweight small risks and are willing to pay far more than expected value to eliminate them altogether. Overweighting of small probabilities increases the attractiveness of both gambles and insurance policies.
The conclusion is straightforward: the decision weights that people assign to outcomes are not identical to the probabilities of these outcomes…
Allais’s Paradox
We took on the task of developing a psychological theory that would describe the choices people make, regardless of whether they are rational. In prospect theory, decision weights would not be identical to probabilities.
Eu: Aqui fica a dúvida: O axioma de racionalidade de von Neuman and Mogerstern está errado??? Ou A Teoria do Prospecto é somente um modelo matemático para calcular como os seres humanos irracionais tomam decisões???
The Fourfold Pattern
When Amos and I began our work on prospect theory, we quickly reached two conclusions: people attach values to gains and losses rather than to wealth, and the decision weights that they assign to outcomes are different from probabilities.
Many unfortunate human situations unfold in the top right cell. This is where people who face very bad options take desperate gambles, accepting a high probability of making things worse in exchange for a small hope of avoiding a large loss. Risk taking of this kind often turns manageable failures into disasters. The thought of accepting the large sure loss is too painful, and the hope of complete relief too enticing, to make the sensible decision that it is time to cut one’s losses. This is where businesses that are losing ground to a superior technology waste their remaining assets in futile attempts to catch up. Because defeat is so difficult to accept, the losing side in wars often fights long past the point at which the victory of the other side is certain, and only a matter of time.
Consider a large organization, the City of New York, and suppose it faces 200 “frivolous” suits each year, each with a 5% chance to cost the city $1 million. Suppose further that in each case the city could settle the lawsuit for a payment of $100,000.
When you take the long view of many similar decisions, you can see that paying a premium to avoid a small risk of a large loss is costly. A similar analysis applies to each of the cells of the fourfold pattern: systematic deviations from expected value are costly in the long run—and this rule applies to both risk aversion and risk seeking. Consistent overweighting of improbable outcomes—a feature of intuitive decision making—eventually leads to inferior outcomes.
Eu: Aqui fica a dúvida: O axioma de racionalidade de von Neuman and Mogerstern está errado?? Ou A Teoria do Prospecto é somente um modelo matemático para calcular como “intuitive decision making” é realizado ???
Chapter 31 - Risk Policies
A rational agent will of course engage in broad framing, but Humans are by nature narrow framers. The combination of loss aversion and narrow framing is a costly curse.
Decision makers who are prone to narrow framing construct a preference every time they face a risky choice. They would do better by having a risk policy that they routinely apply whenever a relevant problem arises. Familiar examples of risk policies are “always take the highest possible deductible when purchasing insurance” and “never buy extended warranties.” A risk policy is a broad frame.
Chapter 32 - Keeping Score
The escalation of commitment to failing endeavors is a mistake from the perspective of the firm but not necessarily from the perspective of the executive who “owns” a floundering project. Canceling the project will leave a permanent stain on the executive’s record, and his personal interests are perhaps best served by gambling further with the organization’s resources in the hope of recouping the original investment—or at least in an attempt to postpone the day of reckoning. In the presence of sunk costs, the manager’s incentives are misaligned with the objectives of the firm and its shareholders, a familiar type of what is known as the agency problem.
Segue um documento do Word com mais considerações sobre essa PART-4 do Livro do Kahneman.
Daniel Kahneman, “Thinking, Fast and Slow”, Farrar, Straus and Giroux, 1st edition (October 1, 2011).
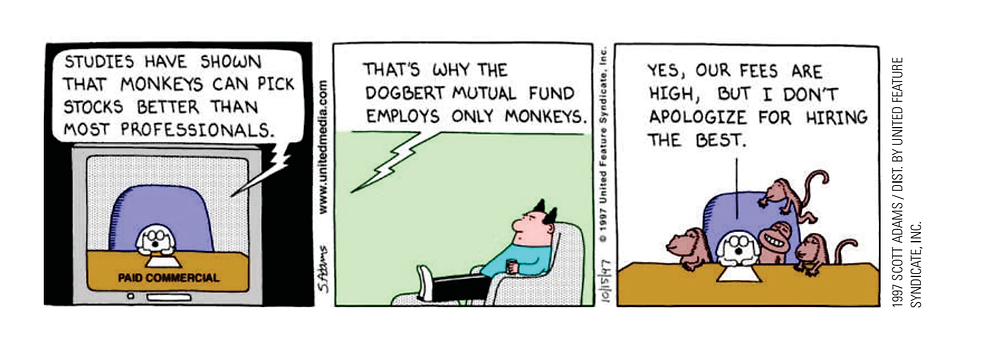
THE ONE ABOUT THE CHIMP
I want to spoil the joke, so I’ll give away the punch line: the average expert was roughly as accurate as a dart-throwing chimpanzee.
You’ve probably heard that one before. It’s famous—in some circles, infamous. It has popped up in the New York Times, the Wall Street Journal, the Financial Times, the Economist, and other outlets around the world. It goes like this: A researcher gathered a big group of experts—academics, pundits, and the like—to make thousands of predictions about the economy, stocks, elections, wars, and other issues of the day. Time passed, and when the researcher checked the accuracy of the predictions, he found that the average expert did about as well as random guessing. Except that’s not the punch line because “random guessing” isn’t funny. The punch line is about a dart-throwing chimpanzee. Because chimpanzees are funny.
I am that researcher (Philip Tetlock) and for a while I didn’t mind the joke. My study was the most comprehensive assessment of expert judgment in the scientific literature. It was a long slog that took about twenty years, from 1984 to 2004, and the results were far richer and more constructive than the punch line suggested. But I didn’t mind the joke because it raised awareness of my research (and, yes, scientists savor their fifteen minutes of fame too). And I myself had used the old “dart-throwing chimp” metaphor, so I couldn’t complain too loudly.
I also didn’t mind because the joke makes a valid point. Open any newspaper, watch any TV news show, and you find experts who forecast what’s coming. Some are cautious. More are bold and confident. A handful claim to be Olympian visionaries able to see decades into the future. With few exceptions, they are not in front of the cameras because they possess any proven skill at forecasting. Accuracy is seldom even mentioned. Old forecasts are like old news—soon forgotten—and pundits are almost never asked to reconcile what they said with what actually happened. The one undeniable talent that talking heads have is their skill at telling a compelling story with conviction, and that is enough. Many have become wealthy peddling forecasting of untested value to corporate executives, government officials, and ordinary people who would never think of swallowing medicine of unknown efficacy and safety but who routinely pay for forecasts that are as dubious as elixirs sold from the back of a wagon. These people—and their customers—deserve a nudge in the ribs. I was happy to see my research used to give it to them.
Noise: How to Overcome
the High, Hidden Cost of Inconsistent Decision Making - Algorithmic judgment is more efficient than the human variety -
Daniel Kahneman, Andrew M. Rosenfield, Linnea Gandhi and Tom Blaser, HBR Magazine, October 2016.